According to foreign media reports, using neurobiological methods, Finnish computer scientists are making new breakthroughs in the process of artificial intelligence research: deep learning neural networks can identify objects in pictures autonomously, with an accuracy rate of 75%.
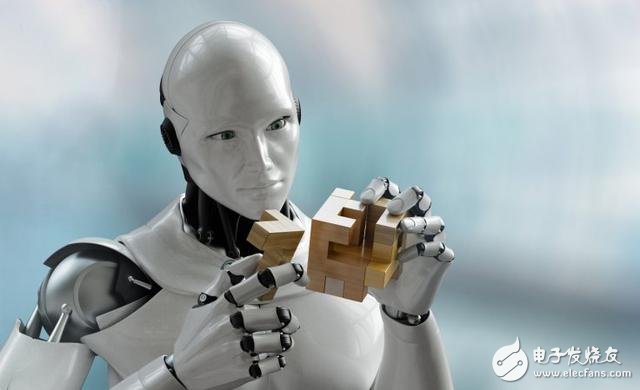
The human brain is very wonderful. After decades of research, humans still can't replicate the ultra-fast computing speed of the human brain. Currently, the most powerful tool that computer scientists can use is neural networks. Such a large computer network can solve complex problems through training, and the mechanism is similar to the human central nervous system, that is, using different levels of neurons to solve different parts of the problem, and finally merged into appropriate answers.
The current problem is that such a neural network requires a lot of data input and training before it can learn how to solve the problem. For example, ImageNet is a good source of training data. This huge visual information database contains 1 million manually labeled photos.
This is called “supervised learning,†and true artificial intelligence means that neural networks need to learn how to automate “unsupervised learningâ€. This is what the Finnish startup Curious AI hopes to achieve.
Curious AI Chief Technology Officer AntTI Rasmus said at the Slush 2016 Technology Industry Conference in Helsinki: "The human brain will do a lot of unsupervised learning. We don't need to tell the baby repeatedly, what is the spoon. They Can automatically learn from the environment and form concepts."
"For the human brain, it is very simple to form a concept based on an object. This has been studied in psychology, that is, 'Gestalt theory.' The human brain will return things with similar shapes, colors, motion states, and patterns. Together, the first step we take is to let the deep learning system classify objects like the human brain."
Applying neuroscience to artificial neural networksIn neuroscience, the theory called "rate coding" suggests that the higher the firing rate of neurons in the brain, the more active the neurons. The neurons continue to be stimulated. In the 1980s, scientists discovered that neurons organize themselves together to represent different information.
This theory is called "temporary coding." The theory holds that the timing of the excitation of neurons is very important, and the exact timing of the excitation defines which neurons belong to the same group in tens of thousands of neurons. Therefore, some neurons can be stimulated at the same time to help the brain recognize an object in a series of objects, such as a red cloth in a pile of office supplies, while another part of the neurons will inform the brain that other objects belong to the background information.
Rasmus said: "Our computer algorithm integrates a temporary encoding mechanism. We save multiple copies in each layer of the neural network. The entire neural network is replicated four times. This means that the system can learn to learn, each The copy represents a specific object, and when these objects are combined, they can be matched with the original image.†Rasmus was a software engineer at Nvidia and is currently working on a PhD study in deep learning at Aalto University in Finland. .
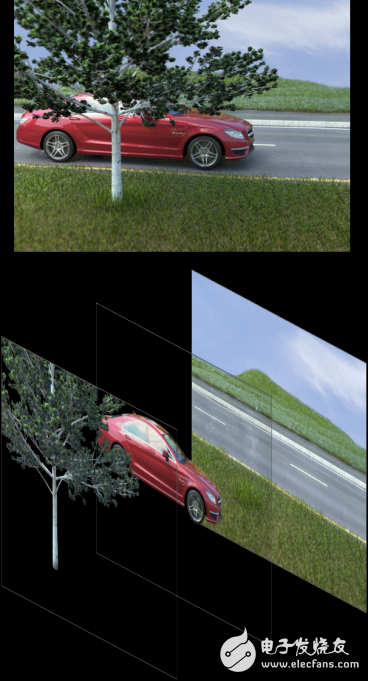
“By dividing the images into four different groups, the neural network can encode the images by itself. This is unsupervised learning, we don't have to mark any system. When we show pictures to the neural network, it breaks down the pictures into elements ( For example, the object in the picture)."
After the neural network breaks down the image into separate elements, it becomes easier to categorize and identify the objects, because the objects do not overlap each other, resulting in blurred images.
Perceptual grouping can bring change to deep learningThe researchers initially directed the neural network to analyze the picture unsupervised, organize the object, and then add tag information (supervised learning) to the image to see what the system learned. They found that Curious AI's Tagger system achieved 75.1% accuracy.
In contrast, the accuracy of traditional neural networks is only 21%, which is only 1% higher than the accuracy of random guesses.
Rasmus said: "This is a revolutionary study, which has led to further development of unsupervised learning. By letting the machine acquire the concept of objects, we have achieved unsupervised learning more similar to the human brain. This can help future research. Let the neural network conduct higher-level reasoning and learn the relevance of the object to the environment."
"In the current system, computers run in a world-based view of statistics. If computers are allowed to enter the world of human life, it is very important to let machines understand the world in a human-like way. It is often difficult to understand the effects of computer vision. Very poor, because human vision is natural to us."
The company's corresponding paper "Tagger: Deep Unsupervised Perception Grouping" will be presented at the "Neuro Information Processing System 2016" Deep Learning Conference in Barcelona on December 7.
Curious AI is looking for industry partners to pilot its deep learning technology in real-world artificial intelligence systems. Currently, the company is in contact with automakers who want to develop driverless technology.
For Samsung Glass,Samsung Galaxy S20 Edge,Samsung Galaxy S20 Ultra Glass,Galaxy S20 Ultra Screen Glass
Dongguan Jili Electronic Technology Co., Ltd. , https://www.touchmanufactor.com